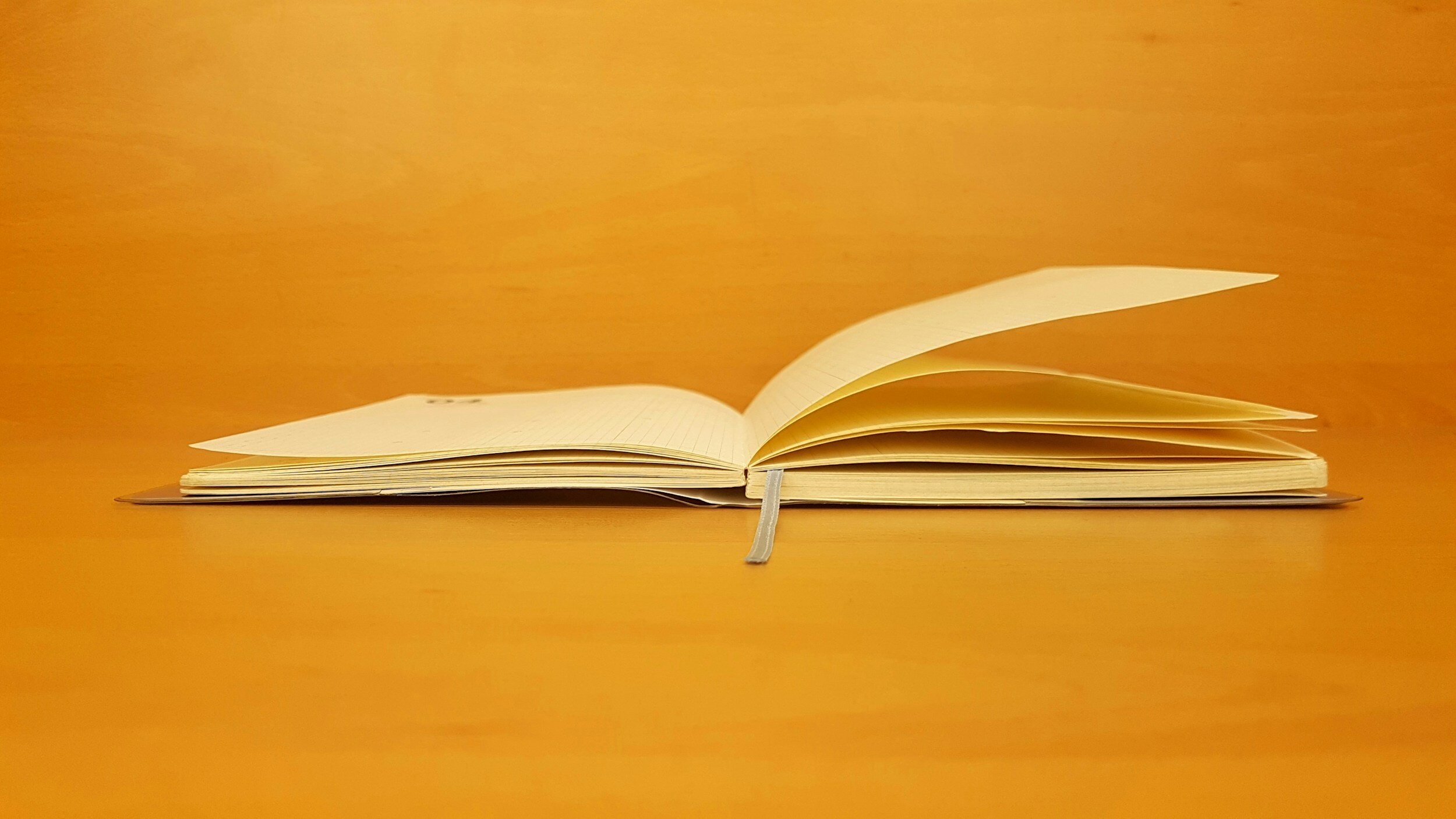
The Marketing AI Glossary
Speak AI. Market Smarter.
Artificial Intelligence (AI): The simulation of human intelligence in machines programmed to think like humans and mimic their actions. In marketing, AI can be used for many use cases, such as content generation, customer segmentation, personalization, predictive analytics, and more.
Big Data: Large and complex data sets that traditional data processing software cannot manage efficiently. Big Data analytics in marketing help in understanding consumer behavior, trends, and making data-driven decisions.
Bias in AI: Refers to systematic and unfair discrimination in the outcomes of AI systems. It's crucial for marketers to understand and address AI bias to ensure ethical practices in targeting, personalization, and customer interaction.
Chatbots: AI programs that simulate human conversation through voice commands, text chats, or both. Chatbots are used in marketing for customer service, engagement, and gathering insights from customer interactions.
Computer Vision: An AI field that trains computers to interpret and understand the visual world. In marketing, computer vision can analyze consumer reactions, track how products are used, or scan social media for brand-related images.
Conversion Rate Optimization (CRO): The process of increasing the percentage of users who perform a desired action on a website. AI tools can analyze user behavior to recommend changes that improve conversion rates.
Customer Segmentation: The process of dividing a customer base into groups of individuals that are similar in specific ways. AI enhances segmentation by analyzing more complex data sets, enabling more personalized marketing strategies.
Data Mining: The process of discovering patterns and knowledge from large amounts of data. Marketers use data mining to extract valuable consumer insights and improve targeting strategies.
Deep Learning: An advanced subset of machine learning that uses neural networks with many layers. It is particularly effective for processing and analyzing large sets of unstructured data, such as images and natural language.
Explainable AI (XAI): The practice of making AI decision-making processes transparent and understandable to humans. For marketers, XAI is important for gaining insights into customer behavior predictions and improving trust in AI recommendations.
Feature Engineering: The process of using domain knowledge to extract features (characteristics, properties, attributes) from raw data that make machine learning algorithms work. For marketers, feature engineering can be crucial in building more effective predictive models.
Generative Adversarial Networks (GANs): A class of AI algorithms used in unsupervised machine learning, implemented by a system of two neural networks contesting with each other. In marketing, GANs can generate realistic images, videos, or voices for creating more engaging content.
Machine Learning (ML): A subset of AI involving the development of algorithms that can learn and make predictions or decisions based on data. Marketers use ML to analyze customer behavior and optimize marketing strategies.
Natural Language Processing (NLP): The ability of computers to understand, interpret, and generate human language. NLP is used in marketing for chatbots, sentiment analysis, and customer service automation.
Personalization: Leveraging data analysis and AI to deliver individualized content to users or customers. In marketing, personalization can increase engagement, conversion rates, and customer satisfaction.
Predictive Analytics: The use of data, statistical algorithms, and ML techniques to identify the likelihood of future outcomes based on historical data. Marketers use predictive analytics for lead scoring, customer lifetime value prediction, and segmentation.
Recommender Systems: A type of information filtering system that seeks to predict the "rating" or "preference" a user would give to an item. In marketing, recommender systems are used extensively in e-commerce for product recommendations, content personalization, and to enhance the overall customer experience.
Reinforcement Learning: A type of ML where an algorithm learns to behave in an environment by performing actions and seeing the results. Marketers might use reinforcement learning to optimize decision-making processes in campaigns.
Semantic Analysis: A part of natural language processing focusing on understanding the meaning and interpretation of words and sentence structure. Marketers use semantic analysis for content optimization, understanding customer feedback, and improving the relevancy of search and recommendations.